Business 2 Nd Puc Introduction To Management
The basic architecture of a data warehouseIn, a data warehouse ( DW or DWH), also known as an enterprise data warehouse ( EDW), is a system used for and, and is considered a core component of. DWs are central repositories of integrated data from one or more disparate sources.
- Business 2 Nd Puc Introduction To Management Business
- 2nd Puc Business Studies Notes In Kannada Pdf
- Introduction To Business Management Pdf
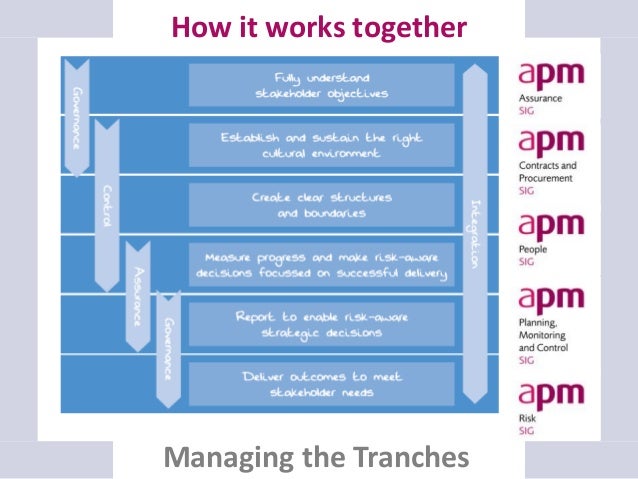
They store current and historical data in one single place that are used for creating analytical reports for workers throughout the enterprise.The data stored in the warehouse is from the (such as marketing or sales). The data may pass through an and may require for additional operations to ensure before it is used in the DW for reporting.The typical (ETL)-based data warehouse uses, and access layers to house its key functions. The staging layer or staging database stores raw data extracted from each of the disparate source data systems. The integration layer integrates the disparate data sets by transforming the data from the staging layer often storing this transformed data in an (ODS) database. The integrated data are then moved to yet another database, often called the data warehouse database, where the data is arranged into hierarchical groups, often called dimensions, and into facts and aggregate facts.
The combination of facts and dimensions is sometimes called a. The access layer helps users retrieve data.The main source of the data is, transformed, catalogued, and made available for use by managers and other business professionals for,. However, the means to retrieve and analyze data, to extract, transform, and load data, and to manage the are also considered essential components of a data warehousing system. Many references to data warehousing use this broader context. Thus, an expanded definition for data warehousing includes, tools to extract, transform, and load data into the repository, and tools to manage and retrieve.
Contents.Benefits A data warehouse maintains a copy of information from the source transaction systems. This architectural complexity provides the opportunity to:. Integrate data from multiple sources into a single database and data model. More congregation of data to single database so a single query engine can be used to present data in an ODS.
Mitigate the problem of database isolation level lock contention in transaction processing systems caused by attempts to run large, long-running, analysis queries in transaction processing databases. Maintain, even if the source transaction systems do not. Integrate data from multiple source systems, enabling a central view across the enterprise. This section needs additional citations for.
Unsourced material may be challenged and removed.Find sources: – ( July 2015) Bottom-up design In the bottom-up approach, are first created to provide reporting and analytical capabilities for specific. These data marts can then be integrated to create a comprehensive data warehouse.
The data warehouse bus architecture is primarily an implementation of 'the bus', a collection of and, which are dimensions that are shared (in a specific way) between facts in two or more data marts. Top-down design The top-down approach is designed using a normalized enterprise., that is, data at the greatest level of detail, are stored in the data warehouse. Dimensional data marts containing data needed for specific business processes or specific departments are created from the data warehouse.
Hybrid design Data warehouses (DW) often resemble the. Feeding the warehouse often include and, generating large amounts of data. To consolidate these various data models, and facilitate the process, data warehouses often make use of an, the information from which is parsed into the actual DW. To reduce data redundancy, larger systems often store the data in a normalized way.
Data marts for specific reports can then be built on top of the data warehouse.A hybrid DW database is kept on to eliminate. A normal relational database, however, is not efficient for business intelligence reports where dimensional modelling is prevalent. Small data marts can shop for data from the consolidated warehouse and use the filtered, specific data for the fact tables and dimensions required. The DW provides a single source of information from which the data marts can read, providing a wide range of business information.
Business 2 Nd Puc Introduction To Management Business
The hybrid architecture allows a DW to be replaced with a repository where operational, not static information could reside.The components follow hub and spokes architecture. This modeling style is a hybrid design, consisting of the best practices from both third normal form. The data vault model is not a true third normal form, and breaks some of its rules, but it is a top-down architecture with a bottom up design. The data vault model is geared to be strictly a data warehouse. It is not geared to be end-user accessible, which when built, still requires the use of a data mart or star schema based release area for business purposes.Data warehouse characteristics There are basic features that define the data in the data warehouse that include subject orientation, data integration, time-variant, nonvolatile data, and data granularity.Subject-Oriented Unlike the operational systems, the data in the data warehouse revolves around subjects of the enterprise.
Subject orientation can be really useful for decision making.Gathering the required objects is called subject oriented.Integrated The data found within the data warehouse is integrated. Since it comes from several operational systems, all inconsistencies must be removed. Consistencies include naming conventions, measurement of variables, encoding structures, physical attributes of data, and so forth.Time-variant While operational systems reflect current values as they support day-to-day operations, data warehouse data represents data over a long time horizon (up to 10 years) which means it stores historical data. It is mainly meant for data mining and forecasting, If a user is searching for a buying pattern of a specific customer, the user needs to look at data on the current and past purchases. Nonvolatile The data in the data warehouse is read-only which means it cannot be updated, created, or deleted. Summarized In the data warehouse, data is summarized at different levels.The user may start looking at the total sale units of a product in an entire region. License key download. Then the user looks at the states in that region.
Finally, they may examine the individual stores in a certain state. Therefore, typically, the analysis starts at a higher level and moves down to lower levels of details. Data warehouse architecture The different methods used to construct/organize a data warehouse specified by an organization are numerous. The hardware utilized, software created and data resources specifically required for the correct functionality of a data warehouse are the main components of the data warehouse architecture. All data warehouses have multiple phases in which the requirements of the organization are modified and fine tuned.
2nd Puc Business Studies Notes In Kannada Pdf
Versus operational system Operational systems are optimized for preservation of and speed of recording of business transactions through use of and an. Operational system designers generally follow of to ensure data integrity.
Introduction To Business Management Pdf
Fully normalized database designs (that is, those satisfying all Codd rules) often result in information from a business transaction being stored in dozens to hundreds of tables. Are efficient at managing the relationships between these tables.
The databases have very fast insert/update performance because only a small amount of data in those tables is affected each time a transaction is processed. To improve performance, older data are usually periodically purged from operational systems.Data warehouses are optimized for analytic access patterns.
Analytic access patterns generally involve selecting specific fields and rarely if ever 'select.' as is more common in operational databases.
Because of these differences in access patterns, operational databases (loosely, OLTP) benefit from the use of a row-oriented DBMS whereas analytics databases (loosely, OLAP) benefit from the use of a.